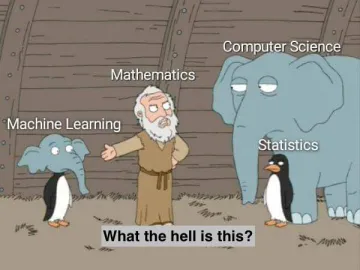
Machine Learning has become one of the main tools to enhance modern data analysis and to constrain models from said data. In cosmology and galaxy formation, machine learning is used in a variety of aspects e.g., to classify galaxies and to measure their photometry and shapes, to increase the speed of modeling and inference frameworks, to enhance precision and accuracy of simulations, and to fully forward-model the entire map of observables directly instead of using summary statistics. Members of our lab use ML in several different research areas:
Machine Learning accelerated cosmological inference:
The challenge of balancing accuracy and speed in modeling complex cosmological observables has been a limiting factor already for the current generation of surveys. This problem will increase significantly given the decrease in statistical uncertainty for future datasets and the corresponding increase in model complexity.
Emulating the results of expensive numerical simulations and subsequent complex calculations that underpin the computation of observables as a function of the parameter space has been identified as the most promising path forward. We are developing neural network architectures that are precise, fast, and computationally inexpensive in performing these computations and that will enable the complex analyses using Roman, Rubin, SPHEREx, and DESI data.
Check out the papers below to learn more about our work:
- Neural network based emulation of galaxy power spectrum covariances -- A reanalysis of BOSS DR12 data
- Machine Learning LSST 3x2pt analyses -- forecasting the impact of systematics on cosmological constraints using neural networks
- Attention-based Neural Network Emulators for Multi-Probe Data Vectors Part I: Forecasting the Growth-Geometry split
- Attention-Based Neural Network Emulators for Multi-Probe Data Vectors Part II: Assessing Tension Metrics
Beyond two-point statistics, simulation-based inference:
Due to nonlinear evolution, the density field of late time has significant cosmological information encoded in its higher order moments. Faculty in our lab use ML to model these higher order moments with the ultimate goal to model the density field directly.
Check out the papers below to learn more!
- A forward modeling approach to analyzing galaxy clustering with SimBIG
- SIMBIG: Mock Challenge for a Forward Modeling Approach to Galaxy Clustering
- Map-based cosmology inference with weak lensing -- information content and its dependence on the parameter space
- Bayesian mass mapping with weak lensing data using karmma: Validation with simulations and application to Dark Energy Survey year 3 data