New paper out! Attention-Based Neural Network Emulators for Multi-Probe Data Vectors Part II: Assessing Tension Metrics
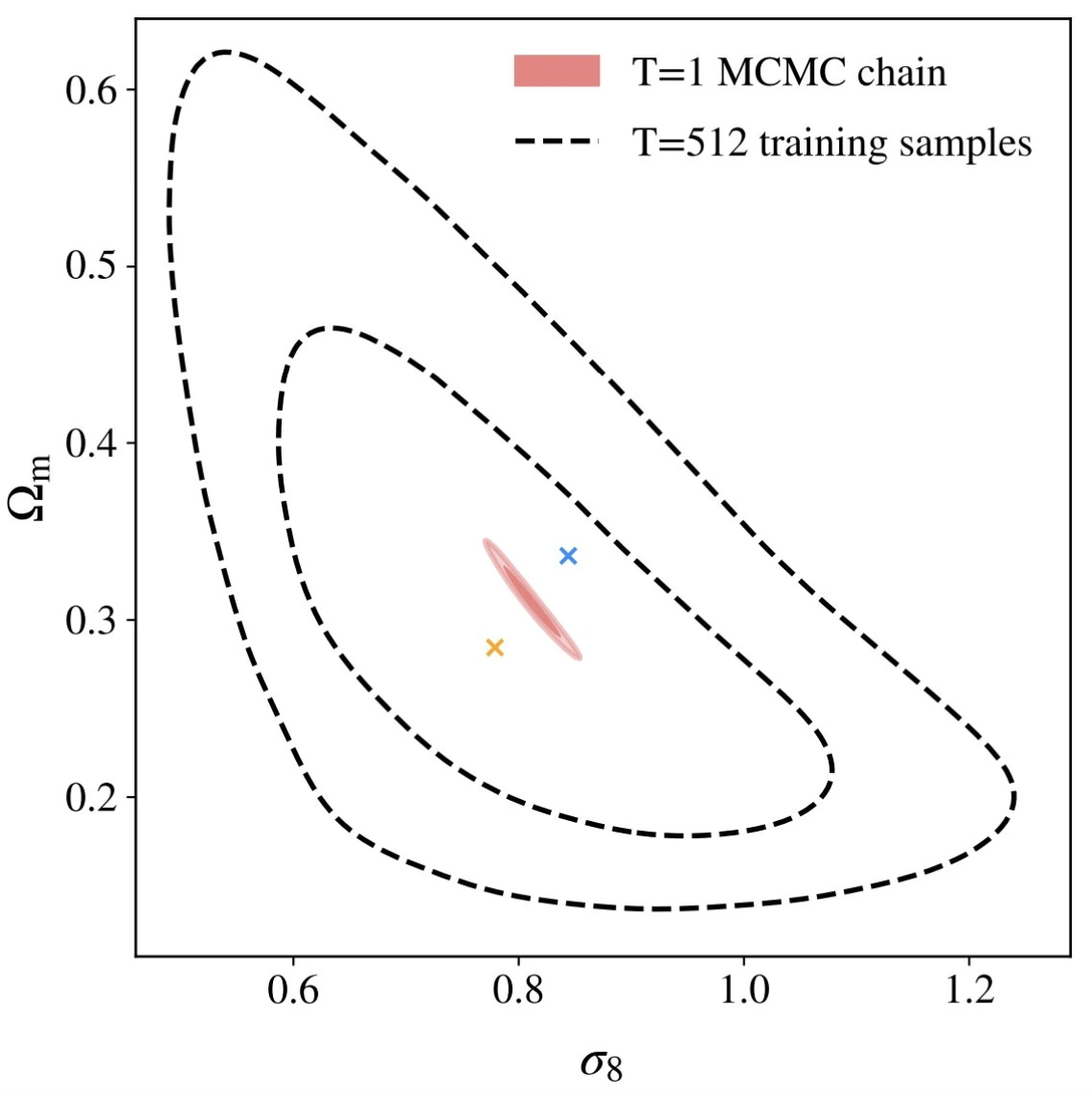
The next generation of cosmological surveys is expected to generate unprecedented high-quality data, consequently increasing the already substantial computational costs of Bayesian statistical methods. This will pose a significant challenge to analyzing theoretical models of cosmology. Additionally, new mitigation techniques of baryonic effects, intrinsic alignment, and other systematic effects will inevitably introduce more parameters, slowing down the convergence of Bayesian analyses. In this scenario, machine-learning-based accelerators are a promising solution, capable of reducing the computational costs and execution time of such tools by order of thousands. Yet, they have not been able to provide accurate predictions over the wide prior ranges in parameter space adopted by Stage III/IV collaborations in studies employing real-space two-point correlation functions. This paper offers a leap in this direction by carefully investigating the modern transformer-based neural network (NN) architectures in realistic simulated Rubin Observatory year one cosmic shear ΛCDM inferences. Building on the framework introduced in Part I, we generalize the transformer block and incorporate additional layer types to develop a more versatile architecture. We present a scalable method to efficiently generate an extensive training dataset that significantly exceeds the scope of prior volumes considered in Part I, while still meeting strict accuracy standards. Through our meticulous architecture comparison and comprehensive hyperparameter optimization, we establish that the attention-based architecture performs an order of magnitude better in accuracy than widely adopted NN designs. Finally, we test and apply our emulators to calibrate tension metrics.