New paper out! Attention-Based Neural Network Emulators for Multi-Probe Data Vectors Part II: Assessing Tension Metrics
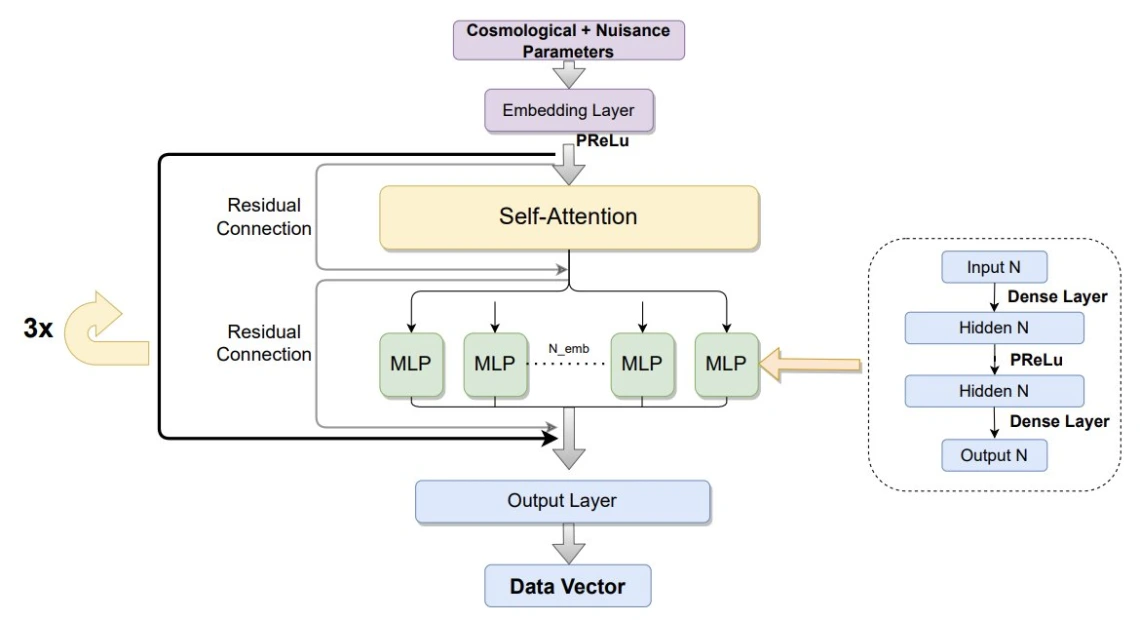
We present a new class of machine-learning emulators that accurately model the cosmic shear, galaxy-galaxy lensing, and galaxy clustering real space correlation functions in the context of Rubin Observatory year one simulated data. To illustrate its capabilities in forecasting models beyond the standard ΛCDM, we forecast how well LSST Year 1 data will be able to probe the consistency between geometry Ωgeom and growth Ωgrowthm dark matter densities in the so-called split ΛCDM parameterization. When trained with a few million samples, our emulator shows uniform accuracy across a wide range in an 18-dimensional parameter space. We provide a detailed comparison of three neural network designs, illustrating the importance of adopting state-of-the-art Transformer blocks. Our study also details their performance when computing Bayesian evidence for cosmic shear on three fiducial cosmologies. The transformers-based emulator is always accurate within PolyChord's precision. As an application, we use our emulator to study the degeneracies between dark energy models and growth geometry split parameterizations. We find that the growth-geometry split remains to be a meaningful test of the smooth dark energy assumption.